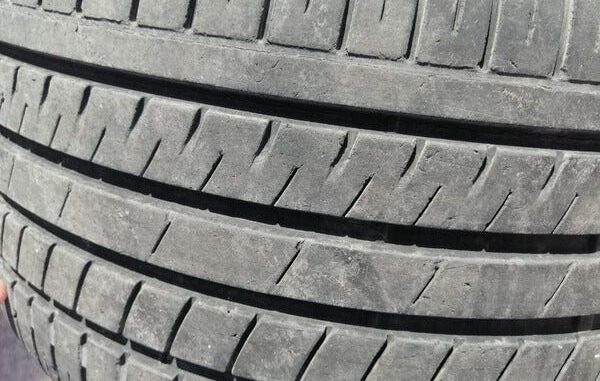
This dataset is a meticulously curated collection of images designed for the automatic detection of vehicle tire conditions. The dataset includes photographs that capture various levels of tire wear under different lighting conditions, making it an ideal resource for training and evaluating machine learning and deep learning models.
- Total Number of Images: 863 Solid Tires 847 Worn Tires
- Total: 1710 Images
- Image Format: JPG
- Average Image Size: 720×720 pixels
- Labels: Solid, Worn
The images were captured under diverse lighting conditions and from different angles to reflect real-world variations. Solid tires were selected for their proper tread depth and absence of deformities, representing ideal operational conditions. In contrast, worn tires show significant wear, such as reduced tread depth, cracks, and deformities, demonstrating varying degrees of degradation.
This dataset serves a wide range of applications, including but not limited to:
- Deep Learning Projects:
- Training models for tire condition detection using Convolutional Neural Networks (CNNs).
2. Machine Learning Studies:
- Performance evaluation with various machine learning models and algorithms.
3. Mobile Application Development:
- Integration into mobile applications for detecting the condition of vehicle tires.
- Optimization: This dataset has been optimized for use in the development and testing of models trained with TensorFlow and Keras. It is also suitable for integration with TensorFlow Lite (TFLite) for mobile applications.
- Processing: Each image is pre-processed to ensure uniformity, with resizing and normalization applied as necessary.
This dataset is freely available for educational and research purposes. For commercial use or redistribution, please contact the dataset owner. When using this dataset, please credit the following reference:
Reference: Rakhmatullo Ergashev, “Vehicle Tire Condition Detection Dataset,” Kaggle, 2024.
For any questions or feedback, feel free to reach out via the following channels:
Be the first to comment