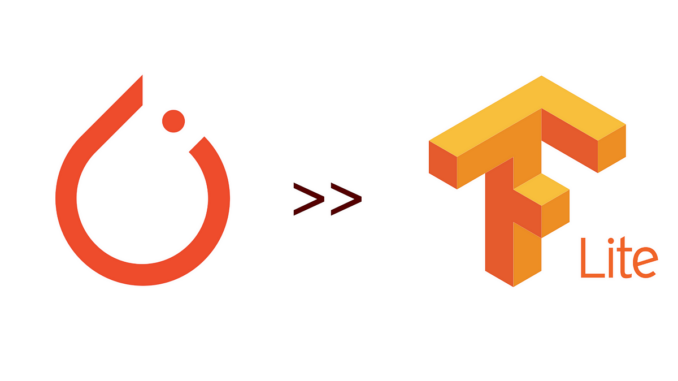
I recently had to convert a deep learning model (a MobileNetV2 variant) from PyTorch to TensorFlow Lite. It was a long, complicated journey, involved jumping through a lot of hoops to make it work. I found myself collecting pieces of information from Stackoverflow posts and GitHub issues. My goal is to share my experience in an attempt to help someone else who is lost like I was.
DISCLAIMER: This is not a guide on how to properly do this conversion. I only wish to share my experience. I might have done it wrong (especially because I have no experience with Tensorflow). If you notice something that I could have done better/differently — please comment and I’ll update the post accordingly.
Convert a deep learning model (a MobileNetV2 variant) from Pytorch to TensorFlow Lite. The conversion process should be:
Pytorch →ONNX → Tensorflow → TFLite
In order to test the converted models, a set of roughly 1,000 input tensors was generated, and the PyTorch model’s output was calculated for each. That set was later used to test each of the converted models, by comparing their yielded outputs against the original outputs, via a mean error metric, over the entire set. The mean error reflects how different are the converted model outputs compared to the original PyTorch model outputs, over the same input.
I decided to treat a model with a mean error smaller than 1e-6 as a successfully converted model.
It might also be important to note that I added the batch dimension in the tensor, even though it was 1. I had no reason doing so other than a hunch that comes from my previous experience converting PyTorch to DLC models.
This was definitely the easy part. Mainly thanks to the excellent documentation on PyTorch, for example here and here.
Requirements:
- ONNX ==1.7.0
- PyTorch ==1.5.1.
The newly created ONNX model was tested on my example inputs and got a mean error of 1.39e-06.
Notice that you will have to convert the torch.tensor
examples into their equivalentnp.array
in order to run it through the ONNX model.
Now that I had my ONNX model, I used onnx-tensorflow (v1.6.0) library in order to convert to TensorFlow. I have no experience with Tensorflow so I knew that this is where things would become challenging.
Requirements:
- tensorflow==2.2.0 (Prerequisite of onnx-tensorflow. However, it worked for me with tf-nightly build
2.4.0-dev20200923
as well) - tensorflow-addons==0.11.2
- onnx-tensorflow == 1.6.0
I’m not sure exactly why, but the conversion worked for me on a GPU machine only.
I ran my test over the TensorflowRep object that was created (examples of inferencing with it here). The run was super slow (around 1 hour as opposed to a few seconds!) so it got me worried. However, eventually, the test produced a mean error of 6.29e-07 so I decided to move on.
The big question at this point was — what was exported? What is this .pb
file?
After some digging online I realized it’s an instance of tf.Graph
. Now all that was left to do is to convert it to TensorFlow Lite.
This is where things got really tricky for me. As I understood it, Tensorflow offers 3 ways to convert TF to TFLite: SavedModel, Keras, and concrete functions. I’m not really familiar with these options, but I already know that what the onnx-tensorflow tool had exported is a frozen graph, so none of the three options helps me 🙁
After quite some time exploring on the web, this guy basically saved my day. It turns out that in Tensorflow v1
converting from a frozen graph is supported! I decided to use v1
API for the rest of my code.
When running the conversion function, a weird issue came up, that had something to do with the protobuf
library. Following this user advice, I was able to move forward.
You would think that after all this trouble, running inference on the newly created tflite
model could be done peacefully. But my troubles did not end there and more issues came up.
One of them had to do with something called “ops “(an error message with “ops that can be supported by the flex.
”). After some digging, I realized that my model architecture required to explicitly enable some operators before the conversion (see above).
Then, it turned out that many of the operations that my network uses are still in development, so the TensorFlow version that was running (2.2.0) could not recognize them. This was solved by installing Tensorflow’s nightly build, specifically tf-nightly==2.4.0.dev20299923
.
Another error I had was “The Conv2D op currently only supports the NHWC tensor format on the CPU. The op was given the format: NCHW
”. This was solved with the help of this user’s comment.
Eventually, this is the inference code used for the tests —
The tests resulted in a mean error of 2.66-07
I hope that you found my experience useful, good luck!
Official Documentation:
Issues and Stackoverflow
Be the first to comment