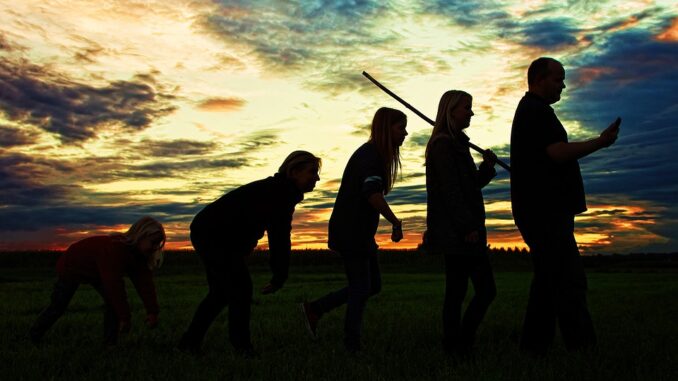
Financial data science has come a long way since its inception. From simply describing the past performance of financial markets and instruments, it has evolved into a highly sophisticated field that uses predictive analytics to forecast future trends and events.
The field of financial data science has its roots in traditional statistics and economics, where analysts would simply gather historical data and use descriptive analytics to understand patterns and trends. However, with the increasing availability of data and the advancement of technology, financial data science has evolved to incorporate predictive analytics, which uses statistical models and machine learning algorithms to forecast future outcomes.
One of the key driving forces behind this evolution has been the exponential growth of data. With the advent of big data, financial institutions and companies have access to large volumes of structured and unstructured data, including market data, customer transaction data, social media data, and more. This abundance of data has provided an opportunity for financial data scientists to delve deeper into predictive analytics and develop models that can anticipate market movements, customer behavior, and other financial events.
Another factor that has contributed to the evolution of financial data science is the advancement of technology. With the development of more powerful computing systems and sophisticated algorithms, financial data scientists are now able to process, analyze, and model large datasets with greater ease and accuracy. This has allowed for the creation of complex predictive models that can generate actionable insights and support decision-making processes.
Furthermore, the need for more proactive and forward-looking financial strategies has also driven the shift towards predictive analytics in financial data science. With the increasing complexity and volatility of financial markets, traditional descriptive analytics are no longer sufficient for making informed decisions. Instead, financial institutions and companies are seeking predictive models that can help them anticipate and adapt to changing market conditions and customer behavior.
As a result, financial data science has become an integral part of decision-making processes within financial institutions and companies. Predictive analytics is now being used to forecast stock prices, identify credit risks, optimize trading strategies, and predict customer churn, among other applications.
In conclusion, the evolution of financial data science from descriptive to predictive analytics has revolutionized the way financial institutions and companies approach data analysis and decision-making. With the incorporation of predictive models and algorithms, financial data science has the potential to revolutionize the way financial markets and institutions operate, making them more agile, efficient, and responsive to changes in the market. As technology continues to advance and data becomes even more abundant, we can expect further advancements in the field of financial data science, bringing with it new opportunities and challenges for analysts and decision-makers in the financial industry.
Be the first to comment