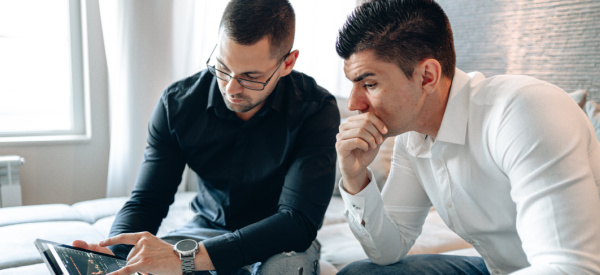
I have previously written that marketers should know (at least) one basic statistical method to properly perform A/B testing. Hopefully, with some knowledge under your belt, you’ll stop letting statistics anxiety hold you back your marketing career and take on a slightly more challenging endeavor: A/B/C Testing.
WHAT IS A/B/C TESTING?
A/B/C testing, much like A/B testing, is a form of controlled experiment. In the case of A/B/C, you are testing more than two versions (hence adding the “C” to A/B) of a variable (web page, page element, email etc.). This can be used to compare 3 or more versions of something to determine which version performs better, like sending multiple emails to see which one generates more engagement or using different advertisements to measure click through rates. One common use case is to challenge a standard or control group against multiple variants. For example, testing a current web page against two alternative web page designs to see which design drives more conversions, the original or the two challengers.
As we’ve written before, there are many different tests you can run, including tools that test multiple components at the same time. Today we’re looking at simple A/B/C test, comparing three versions on one measurement. This could be open rates or click-through rates on emails, advertisements, or web pages.
Learn more about classification and regression trees – powerful approaches to solving predictive analytics problems
Introducing Binary Logistic Regression
Binary logistic regression analysis is used to describe the relationship between a set of predictors and a binary response. A binary response has two outcomes, such as pass or fail. In marketing, this often translates into clicks, opens, or conversions. When you are only comparing two approaches, simpler methods exist, like the two-proportion test.
An EXAMPLE OF AN A/B/C TEST
Imagine a marketer runs a regular ad campaign on social media to drive visitors to their website. They decide to run an A/B/C Test with different versions of the advertisement to see which advertisement will drive the most clicks. They target 20,000 impressions for each advertisement and run their test. They collect their results and graph them. Based on the individual value plot, it is clear that Version A performed worse than the Original and Version B. The question remains: are the differences statistically significant to move away from the original?
BINARY LOGISTIC REGRESSION TO ANALYZE THE TEST
With the data collected, I can use Minitab to fit a binary logistic regression model.
By going to Stat > Regression > Binary Logistic Regression > Fit Binary Logistic Model, Minitab presents a dialogue window for me to select “Response in event/trial format,” and fill in my events (clicks) and trials (impressions). I also select Advertisement as the item I’m testing and let Minitab build my model!
CONCLUSIONS FROM ANAlyzing The RESULTS
Now we need to dive into a little bit of statistics (not much, just a little! You are here to learn something aren’t you?). Looking at the table below, we see the Odds Ratio which compares the odds of two events, in our case clicking on the different advertisements. Minitab sets up the comparison by listing the levels in 2 columns, Level A and Level B. Level B is the reference level for the factor. Odds ratios that are greater than 1 indicate that the event, in our case clicks, is more likely at level A. Odds Ratios that are less than 1 indicate that there is less likely to be a click at Level A.
With regards to our table, comparing Version A to the Original, an Odds Ratio less than 1 means that a click is less likely to happen at Version A. Going down the table, we see that Version B is more likely to get a click than both the original and Version A. This validates what we graphed and compared, but where is the additional information?
By looking at the second column, 95% Confidence Interval, we gain additional insight into our data. In these types of analyses, Confidence Intervals that contain 1 within their range (like Version B versus the Original where the 95% CI is 0.9882,1.1038) indicate the odds of a click vs no click are essentially the same for the two groups.
As a result, this test has taught us that, without question, Version A is the worst performing advertisement and not worth keeping around. However, it would be a mistake to automatically substitute Version B for the original. Our next steps should be to either a) refine our test to an A/B test comparing the Original to Version B or b) select the Original or Version B for qualitative reasons like “sticking with our consistent messaging” or “refreshing our messaging” without worrying about compromising results.
Be the first to comment