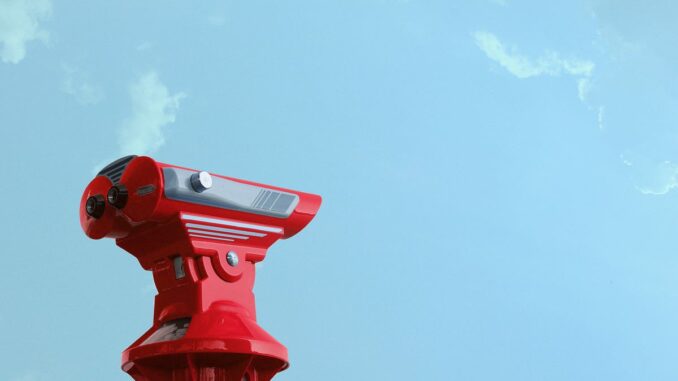
New exciting ideas that marry causality with generative modeling, conformal prediction and topology.
NeurIPS is considered one of the most important and prestigious conferences on artificial intelligence and machine learning globally due to its rigorous paper review process and high quality of accepted research.
With its multidisciplinary focus, the conference covers an extensive range of topics related to developing intelligent systems and machine learning algorithms.
The number of causality-related papers accepted at NeurIPS grew exponentially over the last couple of years.
In this article we introduce five causal papers accepted at the 2023 edition of the conference that drew my attention as bringing important new insights to the field.
Note that this is a subjective and certainly an incomplete list. One of the reasons for this is that at the time of writing NeurIPS still hasn’t published the full list of papers accepted at the conference.
That said, I am convinced that the ideas presented in the papers introduced below have a chance to move our field forward.
Let’s start!
Conformal prediction is a family of uncertainty quantification techniques originally proposed by Vladimir Vovk.
Conformal prediction is model-free (no distributional assumptions are needed) and provides frequentist coverage guarantees. In other words, it guarantees that the true outcome will fall into the prediction intervals (or sets) with high probability under the exchangeability assumption¹.
In their new paper Conformal Meta-learners for Predictive Inference of Individual Treatment Effects just accepted at NeurIPS 2022, Ahmed Alaa and colleagues propose a new framework for conformal meta-learners.
Their approach enables direct inference on the target parameter (individualized treatment effect; ITE), an…
Be the first to comment