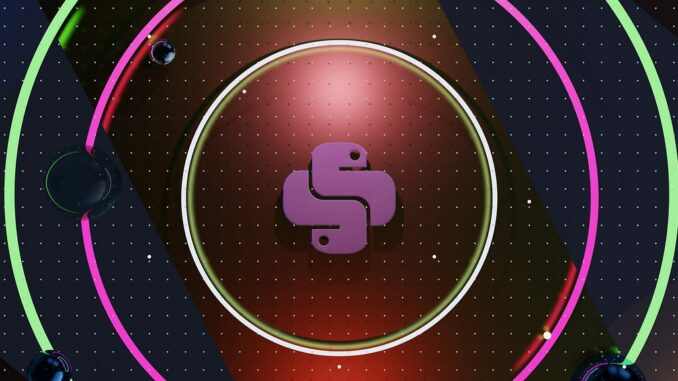
In TensorFlow, you’ll often work with various functions and methods to perform tasks related to machine learning and deep learning. Here are some important functions and methods commonly used in TensorFlow:
- tf.constant(): Creates a constant tensor with a specified value.
import tensorflow as tf
constant_tensor = tf.constant([1, 2, 3])
- tf.Variable(): Creates a mutable tensor variable.
variable = tf.Variable([1.0, 2.0, 3.0])
- tf.placeholder(): Deprecated in TensorFlow 2.x. Used to define placeholders for input data in TensorFlow 1.x.
- tf.keras.layers(): A collection of layers for building neural networks using the Keras API integrated into TensorFlow.
model = tf.keras.Sequential([tf.keras.layers.Dense(64, activation='relu', input_shape=(784,)), tf.keras.layers.Dropout(0.2), tf.keras.layers.Dense(10, activation='softmax') ])
- tf.optimizers(): A collection of optimization algorithms for training neural networks.
optimizer = tf.optimizers.Adam(learning_rate=0.001)
- tf.losses(): A collection of loss functions for training neural networks.
loss_fn = tf.losses.MeanSquaredError()
- tf.data.Dataset(): Used for efficient data input pipelines, helping load and preprocess data.
dataset = tf.data.Dataset.from_tensor_slices((x_train, y_train)
- tf.GradientTape(): Used for automatic differentiation to compute gradients during training.
with tf.GradientTape() as tape:
predictions = model(inputs)
loss = loss_fn(labels, predictions)
gradients = tape.gradient(loss, model.trainable_variables)
- model.compile(): Configures the model for training by specifying the optimizer, loss, and metrics.
model.compile(optimizer='adam', loss='sparse_categorical_crossentropy', metrics=['accuracy'])
model.fit(): Trains the model on a dataset.
model.fit(x_train, y_train, epochs=10, validation_data=(x_val, y_val))
model.evaluate(): Evaluates the model’s performance on a dataset.
loss, accuracy = model.evaluate(x_test, y_test)
tf.saved_model.save(): Saves the model in the SavedModel format for deployment.
tf.saved_model.save(model, "saved_model")
tf.keras.callbacks(): A collection of callback functions for monitoring and customizing training.
pythoncallbacks = [
tf.keras.callbacks.EarlyStopping(patience=5),
tf.keras.callbacks.ModelCheckpoint(filepath='model.h5', save_best_only=True)
]
These are just a few of the important functions and methods used in TensorFlow. TensorFlow’s extensive library provides tools for building and training complex machine learning models, making it a versatile platform for a wide range of deep learning tasks.
Be the first to comment