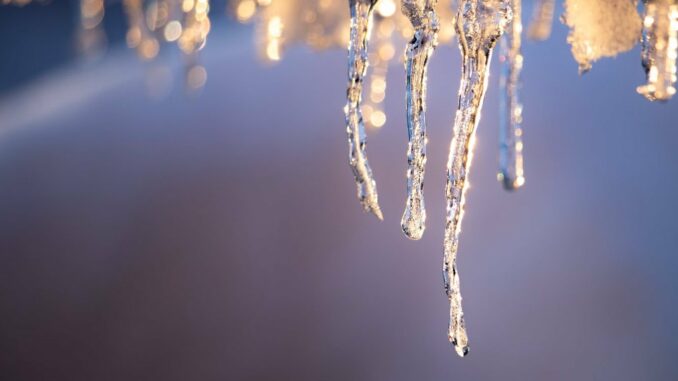
There are few things worse than a cold house or apartment.
Living in Chicago, I’ve come to know the struggle all too well, and let me tell you, it is unpleasant.
To give a bit of background information, I live in a duplex-up condo in Chicago. We have a back room which serves as half guest room, half office. So, when I work remotely or outside standard hours, that’s where I do most of my work for Minitab.
There is one problem, though. The room is cold. Not just a little bit cold, but very cold. There are two heating vents, but the room is not insulated very well (three of the walls are also outside walls) and there is a large double door which leads to our back deck and two large windows.
One day in December, I decided that enough was enough. I wanted to better understand why our home office was so cold.
Luckily, I have access to Minitab’s full suite of solutions.
methods
First, I needed to decide which variables could possibly impact the temperature in our office. After doing some research, I landed on the outside temperature, the wind speed, whether the door to the hallway was open or closed and whether our downstairs neighbors were in town since they generally turn their thermostat down while traveling. I hypothesized that leaving the hallway door open might allow more heat to enter the room.
From December 28th through January 16th, I recorded all the variables at roughly the same time every day—6:00PM. I also used an Ecobee thermometer to record the inside temperature of the office to see which variables had the biggest impact. I set our thermostat at 70 degrees each day to reduce variation.
Then, I ran the numbers.
Results
It’s not a secret that I’m not a statistician, so it’s a good thing that Minitab Statistical Software and Predictive Analytics are so easy to use.
After using Minitab’s Predictive Analytics module, I came away with several key findings. I used Automated Machine Learning to generate a relative variable importance chart and one predictor partial dependence plots.
First, with the relative variable importance chart I was able to determine that the two most important variables regarding the temperature in the office were the status of the hallway door being open and the outside wind speed:
While my neighbors being in town and the outside temperature did still have a slight impact, it was much less than the door and the wind. The one predictor partial dependence plot further shows how as the wind speed was higher, the room was colder:
I made the not-so-crazy leap that the insulation on the windows and doors may have something to do with this disparity.
I went to Home Depot and purchased a couple window insulation kits and one door kit. I installed them that night. I also started consistently leaving the door to the interior hallway open at night and during the day (when possible) to see if that intervention helped.
I continued to record the inside temperature for several days, but immediately noticed a drastic difference—the mean temperature jumped up over 10 degrees, as seen with Minitab’s descriptive statistics:
Temperature before interventions.
Temperature after interventions.
Further, you can visualize this difference with this box plot which measures the interior temperatures before I added weatherproofing to the windows and door and after:
This confirmed that the variables I addressed were indeed the two most important factors making the room so cold. And, while I can’t control the wind speed, I was able to take practical steps to make improvements.
Takeaways
Data is all around us. Before working for Minitab, I would not have thought about harnessing data analysis to solve this unpleasant problem. And I probably would still be cold.
Most data is collected and never analyzed. When faced with decision-making, it’s crucial to be data-driven, whether you’re addressing challenges like keeping your feet warm while working, reducing raw materials in manufacturing or even determining the ideal combination of variables for creating a highly-effective vaccine. The insights gained from your analysis can then lead to simple, concrete steps that save you time and money.
In this instance, I was able to save considerable resources. Instead of cranking up the heat and using more energy and costs, I can keep my office comfortable at a lower, consistent temperature through making data-driven improvements.
Based on my research, this environmentally friendly change should save me about 7-9% on my monthly energy bill, all thanks to the power of data.
Ready to Try Minitab? Download a Free Trial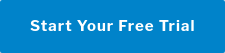
Be the first to comment