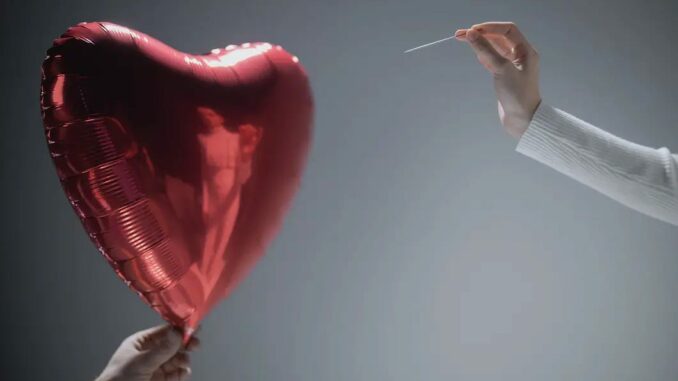
My hope is that by the end of this article you will have a good understanding of how philosophical thinking around causation applies to your work as a data scientist. Ideally you will have a deeper philosophical perspective to give context to your work!
This is the third part in a multi-part series about philosophy and data science. Part 1 covers how the theory of determinism connects with data science and part 2 is about how the philosophical field of epistemology can help you think critically as a data scientist.
Introduction
I love how many philosophical topics take a seemingly obvious concept, like causality, and make you realize it is not as simple as you think. For example, without looking up a definition, try to define causality off the top of your head. That is a difficult task — for me at least! This exercise hopefully nudged you to realize that causality isn’t as black and white as you may have thought.
Here is what this article will cover:
- Challenges of observing causality
- Deterministic vs probabilistic causality
- Regularity theory of causality
- Process theory of causality
- Counterfactual theory of causality
- Bringing it all together
Causality’s Unobservability
David Hume, a famous skeptic and one of my favorite philosophers, made the astute observation that we cannot observe causality directly with our senses. Here’s a classic example: we can see a baseball flying towards the window and we can see the window break, but we cannot see the causality directly. We cannot…
Be the first to comment