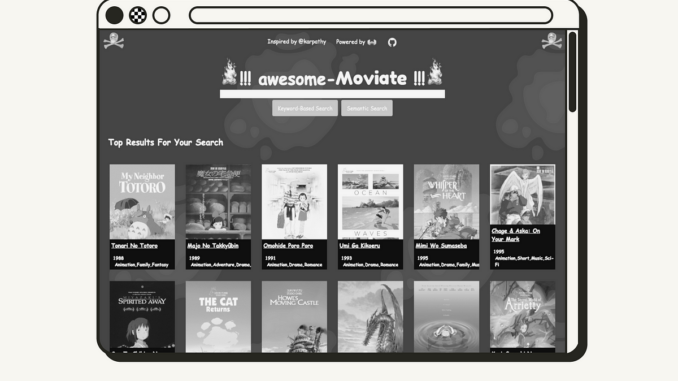
To enable keyword-based search, you can use a .withBm25()
search query across the properties ['title', 'director', 'genres', 'actors', 'keywords', 'description', 'plot']
. You can give the property 'title'
a bigger weight by specifying 'title^3'
.
async function get_keyword_results(text) {
let data = await client.graphql
.get()
.withClassName('Movies')
.withBm25({query: text,
properties: ['title^3', 'director', 'genres', 'actors', 'keywords', 'description', 'plot'],
})
.withFields(['title', 'poster_link', 'genres', 'year', 'director', 'movie_id'])
.withLimit(num_movies)
.do()
.then(info => {
return info
})
.catch(err => {
console.error(err)
})
return data;
}
To enable semantic search, you can use a .withNearText()
search query. This will automatically vectorize the search query and retrieve its closest movies in the vector space.
async function get_semantic_results(text) {
let data = await client.graphql
.get()
.withClassName('Movies')
.withFields(['title', 'poster_link', 'genres', 'year', 'director', 'movie_id'])
.withNearText({concepts: [text]})
.withLimit(num_movies)
.do()
.then(info => {
return info
})
.catch(err => {
console.error(err)
});
return data;
}
To enable hybrid search, you can use a .withHybrid()
search query. The alpha : 0.5
means that keyword search and semantic search are weighted equally.
async function get_hybrid_results(text) {
let data = await client.graphql
.get()
.withClassName('Movies')
.withFields(['title', 'poster_link', 'genres', 'year', 'director', 'movie_id'])
.withHybrid({query: text, alpha: 0.5})
.withLimit(num_movies)
.do()
.then(info => {
return info
})
.catch(err => {
console.error(err)
});
return data;
}
Step 3: Get similar movie recommendations
To get similar movie recommendations, you can do a .withNearObject()
search query, as shown in the queries.js
file. By passing the movie’s id
, the query returns the num_movies = 20
closest movies to the given movie in the vector space.
async function get_recommended_movies(mov_id) {
let data = await client.graphql
.get()
.withClassName('Movies')
.withFields(['title', 'genres', 'year', 'poster_link', 'movie_id'])
.withNearObject({id: mov_id})
.withLimit(20)
.do()
.then(info => {
return info;
})
.catch(err => {
console.error(err)
});
return data;
}
Step 4: Run the demo
Finally, wrap everything up nicely in a web application with the iconic 2000s GeoCities aesthetic (I’m not going to bore you with frontend stuff), and voila! You’re all set!
To run the demo locally, clone the GitHub repository.
git clone git@github.com:weaviate-tutorials/awesome-moviate.git
Navigate to the demo’s directory and set up a virtual environment.
python -m venv .venv
source .venv/bin/activate
Make sure to set the environment variables for your $OPENAI_API_KEY in your virtual environment. Additionally, run the following command in the directory to install all required dependencies in your virtual environment.
pip install -r requirements.txt
Next, set your OPENAI_API_KEY in the docker-compose.yml
file and run the following command to run Weaviate locally via Docker.
docker compose up -d
Once your Weaviate instance is up and running, run the add_data.py
file to populate your vector database.
python add_data.py
Before you can run your application, install all required node modules.
npm install
Finally, run the following command to start up your movie search engine application locally.
npm run start
Now, navigate to http://localhost:3000/ and start playing around with your application.
This article has recreated Andrej Karpathy’s fun weekend project of a movie search engine/recommender system. Below, you can see a short video of the finished live demo:
In contrast to the original project, this project uses a vector database to store the embeddings. Also, the search functionality was extended to allow for semantic and hybrid searches as well.
If you play around with it, you’ll notice that it is not perfect, but just as Karpathy has said:
“it works ~okay hah, have to tune it a bit more.”
You can find the project’s open source code on GitHub and tweak it if you like. Some suggestions for further improvements could be to play around with vectorizing different properties, to tweak the weighting between keyword search and semantic search or to switch out the embedding model with an open source alternative.
Subscribe for free to get notified when I publish a new story.
Be the first to comment