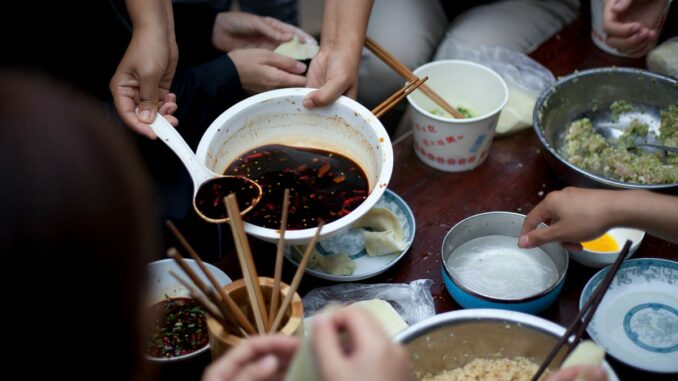
When accessible large language models first came on the scene, the excitement was impossible to miss: beyond their sheer novelty, they came with the promise to completely transform numerous fields and lines of work.
Almost a year after the launch of ChatGPT, we’re far more aware of LLMs’ limitations, and of the challenges we face when we try to integrate them into real-world products. We’ve also, by now, come up with powerful strategies to complement and enhance LLMs’ potential; among these, retrieval-augmented generation (RAG) has emerged as—arguably—the most prominent. It gives practitioners the power to connect pre-trained models to external, up-to-date information sources that can generate more accurate and more useful outputs.
This week, we’ve gathered a potent lineup of articles that explain the intricacies and practical considerations of working with RAG. Whether you’re deep in the ML trenches or approaching the topic from the perspective of a data scientist or product manager, gaining a deeper familiarity with this approach can help you prepare for whatever the future of AI tools brings. Let’s jump right in!
Be the first to comment