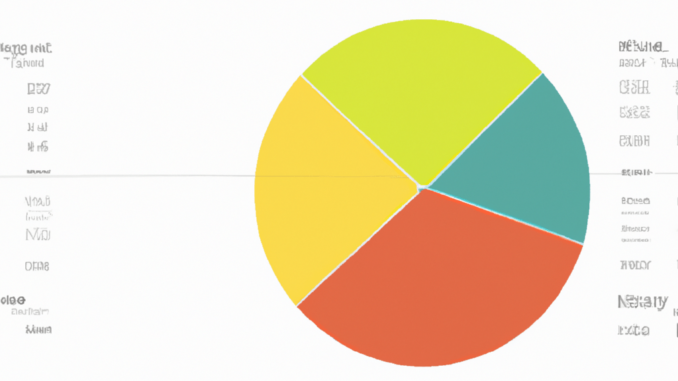
Soha Elghany posed a question on Twitter that stopped me in my tracks. Further in the thread, she brings up the recent award-winning art created in Midjourney.
I love these types of questions. Before going into interpreting, I sought to understand the underlying dynamics of work. Interpreting gave me audience to the way we work, letting me see the sociodynamics of work conversations in a new light. A part of my interpreting degree meant understanding the difference between a practice profession and other trades out there. As CVO of Versalytix, I look at analytics both as a direct contributor to the product, but also as an executive looking to keep my business going and growing.
The pace of innovation establishes our rhythms
Technologies shape our society. They reframe how we work, pivot the skills we value, and feed back into human culture to further inform innovation. I can remember sitting in offices where computers sat heavily framed by paper file folders, the machines a part of the work process, but a small fraction of it. In the early days, we printed everything with multiple copies. I have even written actual memos we posted around the office (sit with that). Eventually, the papers thinned and the rare papers I have laying around are typically printed by someone else, but are most often colorful sticky notes for my kanban board.
Computers inform how I do my work. My contributions to the world exist almost entirely in the digital sphere. Should the electric go out à la Revolution style, I’d have to pivot to something far more tangible like interpreting, massage work, or – dare I say it – attempting to cook.
I wasn’t always this way. I used to remember numbers, memorize price points of fabrics by feel, and could commit book facts to memory. These skills, left dusty by disuse, waned while certain digital navigation skills increased and my proficiency to bend digital tools to my will took priority. Just as the engine transformed travel, digitization alters work thoroughly and completely. Writing by hand versus typing not only affects how we write, but the voice we use. Typing creates a brevity, a terseness at times, that older generations writing by longhand do not share. To channel Marshall McLuhan, the medium shapes message. Further innovations, such as text autocomplete, move closer to scripting what the digital voice says. Can you reject these choices? Absolutely. And those choices will likely provide data in the future.
Throughout the years, the written voice in books changed. The technologies at the time played a role, as well as the frequency of dispatches and cultural transformation. The digital voice, one flung far and wide, demands a different type of prose, one that plays well with translation tools for maximum impact. I can expect at least 20% of readers of this blog to access my words in a language other than English. Some decades earlier, that very technology spurred similar questions for me that Soha has around Ask Data.
Google Translate as a case study
Language translation is hard work. It’s seen as expensive and historically was limited to only certain types of content. Legally, within the US, certain materials must be translated in a handful of languages (usually “top languages” within the area) to provide reasonable access. In hospitals, for example, informed consent documents may appear in 2-3 languages outside of English. Other languages depend on an interpreter to sight-translate (read and interpret verbally) into that language. This type of access limits the control the patient has – they cannot easily reread, skim, and must explicitly ask for repetition while a reader can shift reading speeds.
In the early days, machine translation promised a far more economical way to quickly parse text and deploy it in other languages. Those attempts read rigidly, requiring the end consumer to do a lot of creative stretching for meaning. By 2016, Translate notably refined its translations, with capabilities today drastically improving. A number of human translators may incorporate AI translation tools as part of their process, in part as a means to explore possible representations of concepts, but also as a check for their own work.


In some ways, this is a refinement of the translation bank most larger translation organizations used. Forms, websites, and a number of other documents often use fairly canned verbiage. Translation attempts to hew closely to the established meaning of the document. If you’ve read translated work, you can viscerally feel some of the mismatches from how you’d compose directly in that language. Localization, rather than translation, adapts content entirely for that targeted region.
A number of human translators pivoted from rote translation work (think the sundry of documents) to localization work. It allows them more play in their work, taking intent and exploring what calls to action would work better for a given area. Translation work broadened as a discipline, allowing some translators to take paths into training the machines themselves and others to push into specialties, in addition to “status quo” translation work. More content is being translated than ever before, with a variety of it coming from places not previously leveraging translation work. This blog, for example, runs on its own steam, where I am the lone administrator, editor, and content generator. Readers from all over the globe can access it in part due to AI translation.
Some content providers are working with other community members to translate targeted articles or works into a variety of languages. Other content providers, such as Rosario Guana, are publishing in more than one language. Within the Tableau community, content creators are also creating content in a variety of languages. Tools like Google Translate allow me, as an English speaker (and ASL signer), the ability to get a sense at what types of techniques are landing where.
The ubiquity of Google Translate is a broader reflection of the globalization of our world. It allows us greater discourse. I can read a Tweet in Korean translated by a click of a button and reply in English. While far from perfect, the interactions would have been much harder historically. Democratizing translation tools means more people can intermittently engage with translation. Translation companies, in turn, recognize they must sell services differently and address the gaps in machine translation. Translation work has changed, for sure, but it hasn’t gone away.
The Art of AI Augmentation
In 2000, Chris Stolte and Pat Hanrahan submitted a paper to the IEEE Symposium on Information Visualization around Polaris, a prototype for visualizing large datasets. Marrying the ease of pivot tables with the intelligence of Grammar of Graphics, Polaris quickly moved from a prototype to what we recognize as Tableau today.
In 2003, the world ran on spreadsheets. Large BI implementations remained the dominion of larger organizations. It required an IT department, a field still very much in its teenage years at the time. Tableau smashed all of that, promising to democratize access and say yes in a world that very much lived on no as the primary paradigm. The smart defaults removed the upfront dependency on chart choosers. Instead, adding data onto the canvas changed the chart. Within the BI community, Tableau created a rift, with users from various paradigms panning Tableau fans. Technologies that democratize access threaten existing power structures.
Over 2 decades later, certain core tenets of Tableau remain the same. The marks card still serves as a the primary interface for creating charts, supplemented by Show Me. Innovations through the years furthered the ability to see and understand data – from dashboards to various calculation and feature enrichments allowed users to go beyond charts to entire data ecosystems and products. Users of Tableau today can draw in outside applications through extensions, embed Tableau directly into customer-facing platforms, and create cohesive narratives that drive change through a variety of interaction types.
But charts alone aren’t enough to engage all users with data. Charts abstract patterns and codify them in semantic and perceptual ways to bring forth intent to users. They require a different skill – graphicacy – to interpret the full meaning embedded in the representation, particularly when multiple charts create a cohesive whole. Users new to charts benefit from a variety of streams into the waters. Innovations like Ask Data and Narrative Science (now branded as Data Stories) provide a textual reference to what users are also seeing. The Data Stories feature explicitly translates the visual while Ask Data allows users to use their own words to begin to inquire about data without being skilled in creating charts.

Ask Data is tool designed not for the analyst, but the lay- or non-user of data. It provides a means (text) to inquire about a fact and provides a learning tool in the vocabulary of charts. Asking about trends provides a line chart consistently. Asking about comparisons returns a bar charts. Through analytical dialogue, Ask Data provides a launchpad for learning to converse in charts. Deploying Ask Data in Slack eases the friction, allowing quick curiosities to be solved without leaving other flows.
As analysts (and the sundry list of other titles we can use), we want Tableau (and other tools relevant to our work) to hold pride of place. We know our work provides the insight that moves business drastically. Dashboards are a part of the solution. But like the translators of yore, Ask Data provides an interstitial space between Google and conventional analytics solutions to answer curated data questions. It’s akin to the machine translating forms or rote text.
Will Ask Data make me redundant?
The short answer: Ask Data will certainly eat specific tasks. In the early days, I made a variety of “Bring Your Own (BYO) Chart” solutions that Ask Data hands-down solves better. There’s a number of parameterized pivot style reports that Ask Data may also augment and ideally encourage people to handle through charts. There are some entry level analytical tasks I definitely predict being dispersed to the broader workforce, rather than handled through an analyst.
For those of us doing analytical work, we can expect to take on the harder jobs. Those that can find compelling information and present it in clear ways will continue to stand apart. The work will further fragment. I’m seeing data team shift from one person handling the entire lifecycle to segmentation into how data is handled, explored (analyst, but also intersecting further with data science), communicated (various front end titles), and introduced to users (Center of Excellence roles). We’re adding nuance to data teams that include UI, UX, and information architecture as fully separate domains and including other roles that explore the interplay through folklore and sociology.
Yet, it’s not only AI and ML that alter the course of our work. It’s also values themselves. Companies that prize innovation will likely invest more both in tools and people. Some brands will push as far as they can with mostly AI solutions augmented by some people – roles in these spaces will absolutely be marked redundant as the technology covers more familiar ground. We’ll see greater distance between the companies that use both types of resources well versus those who pivot solely to AI – human ingenuity expands what’s feasible and finds new insight.

While Midjourney created a stunning picture of what’s possible, a human still found what made it beautiful. Just like photography disrupted how much we value photorealistic painting (well illustrated by discourse around former US President Obama’s latest portrait), technology transforms what we see as innovative. Ask Data will transform the work we do, even if we as designers and architects of dashboards never use it directly. Embedded in certain dashboards, it provides that point of clarification that would be handled either as a one-off or an interation that slowly snowballs a certain dashboard far beyond a useful scope.
The broader question about the future of work remains wildly open. In 2005, Daniel Pink noted what helped humans stand apart from machines was storytelling. Others, such as former Tableau CEO Christian Chabot pinned it on curiousity and creativity. Technology will continue to force us to define what makes us stand out.
As technology continues to disrupt and displace broader swaths of the workforce, we are going to have to redefine what working as a society means. Countries will determine their own answers, from universal basic income or altering expectations around what makes a workweek to how much productivity to pursue. Few people within the recent few generations can expect to hold the same job they have now. Career transformation is already the norm in many countries and this will likely continue for the foreseeable future. I don’t see analysis vanishing, but I definitely predict it will change.
Be the first to comment