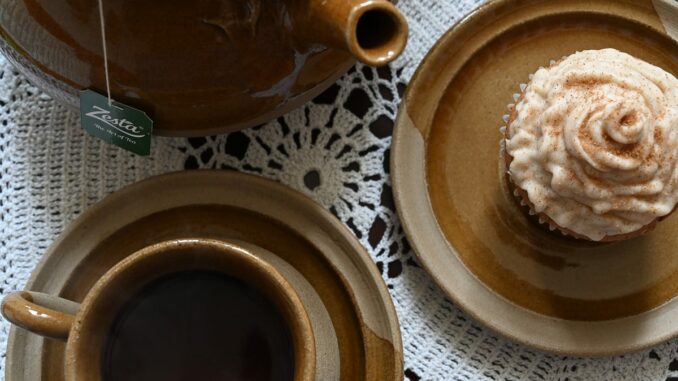
Getting a handle on the current state of machine learning is tricky: on the one hand, it takes time to catch up with foundational concepts and methods, even if you’ve worked in the field for a while. On the other hand, new tools and models keep popping up at a rapid clip. What’s an ML learner to do?
We tend to favor a balanced, cumulative approach—one that recognizes that no single person can master all the knowledge out there, but that digesting well-scoped pieces of information at a steady, ongoing cadence will help you gain a firm footing in the field.
Our selection of highlights this week reflects that belief: we’ve chosen a few well-executed articles that cover both essential topics and cutting-edge ones, and that both beginners and more seasoned professionals can benefit from reading. Let’s dive in.
- DINO — A Foundation Model for Computer Vision
If you learn best by diving deep into a topic, you don’t want to miss Sascha Kirch’s series, which unpacks and contextualizes influential machine learning papers, one model at a time. In a recent installation, Sascha walked us through the inner workings of DINO, a foundation model based on the groundbreaking abilities of visual transformers (ViT). - Exploring GEMBA: A New LLM-Based Metric for Translation Quality Assessment
Machine translation isn’t exactly a novel technology, but the rise of LLMs has generated new possibilities for enhancing current tools and workflows. Dr. Varshita Sher’s latest article introduces us to GEMBA, a recently introduced metric that leverages the power of GPT models to evaluate the quality of machine-translated text. - Machine Learning, Illustrated: Incremental Learning
For the visual learners out there, and especially those taking their first steps in the field, Shreya Rao’s beginner-friendly guide to incremental learning addresses a key question: how do models maintain and build upon existing knowledge?
Be the first to comment